Classes |
Public Member Functions |
Protected Member Functions |
Protected Attributes |
List of all members
ompl::geometric::RRTstar Class Reference
Optimal Rapidly-exploring Random Trees. More...
#include <ompl/geometric/planners/rrt/RRTstar.h>
Inheritance diagram for ompl::geometric::RRTstar:
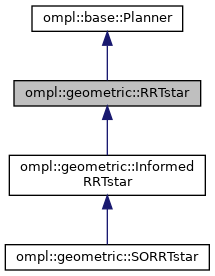
Classes | |
struct | CostIndexCompare |
class | Motion |
Representation of a motion. More... | |
Public Member Functions | |
RRTstar (const base::SpaceInformationPtr &si) | |
void | getPlannerData (base::PlannerData &data) const override |
Get information about the current run of the motion planner. Repeated calls to this function will update data (only additions are made). This is useful to see what changed in the exploration datastructure, between calls to solve(), for example (without calling clear() in between). | |
base::PlannerStatus | solve (const base::PlannerTerminationCondition &ptc) override |
Function that can solve the motion planning problem. This function can be called multiple times on the same problem, without calling clear() in between. This allows the planner to continue work for more time on an unsolved problem, for example. If this option is used, it is assumed the problem definition is not changed (unpredictable results otherwise). The only change in the problem definition that is accounted for is the addition of starting or goal states (but not changing previously added start/goal states). If clearQuery() is called, the planner may retain prior datastructures generated from a previous query on a new problem definition. The function terminates if the call to ptc returns true. | |
void | clear () override |
Clear all internal datastructures. Planner settings are not affected. Subsequent calls to solve() will ignore all previous work. | |
void | setup () override |
Perform extra configuration steps, if needed. This call will also issue a call to ompl::base::SpaceInformation::setup() if needed. This must be called before solving. | |
void | setGoalBias (double goalBias) |
Set the goal bias. More... | |
double | getGoalBias () const |
Get the goal bias the planner is using. | |
void | setRange (double distance) |
Set the range the planner is supposed to use. More... | |
double | getRange () const |
Get the range the planner is using. | |
void | setRewireFactor (double rewireFactor) |
Set the rewiring scale factor, s, such that r_rrg = s \times r_rrg* (or k_rrg = s \times k_rrg*) | |
double | getRewireFactor () const |
Set the rewiring scale factor, s, such that r_rrg = s \times r_rrg* > r_rrg* (or k_rrg = s \times k_rrg* > k_rrg*) | |
template<template< typename T > class NN> | |
void | setNearestNeighbors () |
Set a different nearest neighbors datastructure. | |
void | setDelayCC (bool delayCC) |
Option that delays collision checking procedures. When it is enabled, all neighbors are sorted by cost. The planner then goes through this list, starting with the lowest cost, checking for collisions in order to find a parent. The planner stops iterating through the list when a collision free parent is found. This prevents the planner from collision checking each neighbor, reducing computation time in scenarios where collision checking procedures are expensive. | |
bool | getDelayCC () const |
Get the state of the delayed collision checking option. | |
void | setTreePruning (bool prune) |
Controls whether the tree is pruned during the search. This pruning removes a vertex if and only if it and all its descendents passes the pruning condition. The pruning condition is whether the lower-bounding estimate of a solution constrained to pass the the vertex is greater than the current solution. Considering the descendents of a vertex prevents removing a descendent that may actually be capable of later providing a better solution once its incoming path passes through a different vertex (e.g., a change in homotopy class). | |
bool | getTreePruning () const |
Get the state of the pruning option. | |
void | setPruneThreshold (const double pp) |
Set the fractional change in solution cost necessary for pruning to occur, i.e., prune if the new solution is at least X% better than the old solution. (e.g., 0.0 will prune after every new solution, while 1.0 will never prune.) | |
double | getPruneThreshold () const |
Get the current prune states percentage threshold parameter. | |
void | setPrunedMeasure (bool informedMeasure) |
Use the measure of the pruned subproblem instead of the measure of the entire problem domain (if such an expression exists and a solution is present). Currently the only method to calculate this measure in closed-form is through a informed sampler, so this option also requires that. | |
bool | getPrunedMeasure () const |
Get the state of using the pruned measure. | |
void | setInformedSampling (bool informedSampling) |
Use direct sampling of the heuristic for the generation of random samples (e.g., x_rand). If a direct sampling method is not defined for the objective, rejection sampling will be used by default. | |
bool | getInformedSampling () const |
Get the state direct heuristic sampling. | |
void | setSampleRejection (bool reject) |
Controls whether heuristic rejection is used on samples (e.g., x_rand) | |
bool | getSampleRejection () const |
Get the state of the sample rejection option. | |
void | setNewStateRejection (const bool reject) |
Controls whether heuristic rejection is used on new states before connection (e.g., x_new = steer(x_nearest, x_rand)) | |
bool | getNewStateRejection () const |
Get the state of the new-state rejection option. | |
void | setAdmissibleCostToCome (const bool admissible) |
Controls whether pruning and new-state rejection uses an admissible cost-to-come estimate or not. | |
bool | getAdmissibleCostToCome () const |
Get the admissibility of the pruning and new-state rejection heuristic. | |
void | setOrderedSampling (bool orderSamples) |
Controls whether samples are returned in ordered by the heuristic. This is accomplished by generating a batch at a time. | |
bool | getOrderedSampling () const |
Get the state of sample ordering. | |
void | setBatchSize (unsigned int batchSize) |
Set the batch size used for sample ordering. | |
unsigned int | getBatchSize () const |
Get the batch size used for sample ordering. | |
void | setFocusSearch (const bool focus) |
A meta parameter to focusing the search to improving the current solution. This is the parameter set by CFOREST. For RRT*, search focusing consists of pruning the existing search and limiting future search. Specifically, this is accomplished by turning on informed sampling, tree pruning and new-state rejection. This flag individually sets the options described above. | |
bool | getFocusSearch () const |
Get the state of search focusing. | |
void | setKNearest (bool useKNearest) |
Use a k-nearest search for rewiring instead of a r-disc search. | |
bool | getKNearest () const |
Get the state of using a k-nearest search for rewiring. | |
void | setNumSamplingAttempts (unsigned int numAttempts) |
Set the number of attempts to make while performing rejection or informed sampling. | |
unsigned int | getNumSamplingAttempts () const |
Get the number of attempts to make while performing rejection or informed sampling. | |
unsigned int | numIterations () const |
ompl::base::Cost | bestCost () const |
![]() | |
Planner (const Planner &)=delete | |
Planner & | operator= (const Planner &)=delete |
Planner (SpaceInformationPtr si, std::string name) | |
Constructor. | |
virtual | ~Planner ()=default |
Destructor. | |
template<class T > | |
T * | as () |
Cast this instance to a desired type. More... | |
template<class T > | |
const T * | as () const |
Cast this instance to a desired type. More... | |
const SpaceInformationPtr & | getSpaceInformation () const |
Get the space information this planner is using. | |
const ProblemDefinitionPtr & | getProblemDefinition () const |
Get the problem definition the planner is trying to solve. | |
ProblemDefinitionPtr & | getProblemDefinition () |
Get the problem definition the planner is trying to solve. | |
const PlannerInputStates & | getPlannerInputStates () const |
Get the planner input states. | |
virtual void | setProblemDefinition (const ProblemDefinitionPtr &pdef) |
Set the problem definition for the planner. The problem needs to be set before calling solve(). Note: If this problem definition replaces a previous one, it may also be necessary to call clear() or clearQuery(). | |
PlannerStatus | solve (const PlannerTerminationConditionFn &ptc, double checkInterval) |
Same as above except the termination condition is only evaluated at a specified interval. | |
PlannerStatus | solve (double solveTime) |
Same as above except the termination condition is solely a time limit: the number of seconds the algorithm is allowed to spend planning. | |
virtual void | clearQuery () |
Clears internal datastructures of any query-specific information from the previous query. Planner settings are not affected. The planner, if able, should retain all datastructures generated from previous queries that can be used to help solve the next query. Note that clear() should also clear all query-specific information along with all other datastructures in the planner. By default clearQuery() calls clear(). | |
const std::string & | getName () const |
Get the name of the planner. | |
void | setName (const std::string &name) |
Set the name of the planner. | |
const PlannerSpecs & | getSpecs () const |
Return the specifications (capabilities of this planner) | |
virtual void | checkValidity () |
Check to see if the planner is in a working state (setup has been called, a goal was set, the input states seem to be in order). In case of error, this function throws an exception. | |
bool | isSetup () const |
Check if setup() was called for this planner. | |
ParamSet & | params () |
Get the parameters for this planner. | |
const ParamSet & | params () const |
Get the parameters for this planner. | |
const PlannerProgressProperties & | getPlannerProgressProperties () const |
Retrieve a planner's planner progress property map. | |
virtual void | printProperties (std::ostream &out) const |
Print properties of the motion planner. | |
virtual void | printSettings (std::ostream &out) const |
Print information about the motion planner's settings. | |
Protected Member Functions | |
void | allocSampler () |
Create the samplers. | |
bool | sampleUniform (base::State *statePtr) |
Generate a sample. | |
void | freeMemory () |
Free the memory allocated by this planner. | |
double | distanceFunction (const Motion *a, const Motion *b) const |
Compute distance between motions (actually distance between contained states) | |
void | getNeighbors (Motion *motion, std::vector< Motion * > &nbh) const |
Gets the neighbours of a given motion, using either k-nearest of radius as appropriate. | |
void | removeFromParent (Motion *m) |
Removes the given motion from the parent's child list. | |
void | updateChildCosts (Motion *m) |
Updates the cost of the children of this node if the cost up to this node has changed. | |
int | pruneTree (const base::Cost &pruneTreeCost) |
Prunes all those states which estimated total cost is higher than pruneTreeCost. Returns the number of motions pruned. Depends on the parameter set by setPruneStatesImprovementThreshold() | |
base::Cost | solutionHeuristic (const Motion *motion) const |
Computes the solution cost heuristically as the cost to come from start to the motion plus the cost to go from the motion to the goal. If the parameter use_admissible_heuristic (setAdmissibleCostToCome()) is true, a heuristic estimate of the cost to come is used; otherwise, the current cost to come to the motion is used (which may overestimate the cost through the motion). | |
void | addChildrenToList (std::queue< Motion *, std::deque< Motion * >> *motionList, Motion *motion) |
Add the children of a vertex to the given list. | |
bool | keepCondition (const Motion *motion, const base::Cost &threshold) const |
Check whether the given motion passes the specified cost threshold, meaning it will be kept during pruning. | |
void | calculateRewiringLowerBounds () |
Calculate the k_RRG* and r_RRG* terms. | |
std::string | numIterationsProperty () const |
std::string | bestCostProperty () const |
![]() | |
template<typename T , typename PlannerType , typename SetterType , typename GetterType > | |
void | declareParam (const std::string &name, const PlannerType &planner, const SetterType &setter, const GetterType &getter, const std::string &rangeSuggestion="") |
This function declares a parameter for this planner instance, and specifies the setter and getter functions. | |
template<typename T , typename PlannerType , typename SetterType > | |
void | declareParam (const std::string &name, const PlannerType &planner, const SetterType &setter, const std::string &rangeSuggestion="") |
This function declares a parameter for this planner instance, and specifies the setter function. | |
void | addPlannerProgressProperty (const std::string &progressPropertyName, const PlannerProgressProperty &prop) |
Add a planner progress property called progressPropertyName with a property querying function prop to this planner's progress property map. | |
Protected Attributes | |
base::StateSamplerPtr | sampler_ |
State sampler. | |
base::InformedSamplerPtr | infSampler_ |
An informed sampler. | |
std::shared_ptr< NearestNeighbors< Motion * > > | nn_ |
A nearest-neighbors datastructure containing the tree of motions. | |
double | goalBias_ {.05} |
The fraction of time the goal is picked as the state to expand towards (if such a state is available) | |
double | maxDistance_ {0.} |
The maximum length of a motion to be added to a tree. | |
RNG | rng_ |
The random number generator. | |
bool | useKNearest_ {true} |
Option to use k-nearest search for rewiring. | |
double | rewireFactor_ {1.1} |
The rewiring factor, s, so that r_rrt = s \times r_rrt* > r_rrt* (or k_rrt = s \times k_rrt* > k_rrt*) | |
double | k_rrt_ {0u} |
A constant for k-nearest rewiring calculations. | |
double | r_rrt_ {0.} |
A constant for r-disc rewiring calculations. | |
bool | delayCC_ {true} |
Option to delay and reduce collision checking within iterations. | |
base::OptimizationObjectivePtr | opt_ |
Objective we're optimizing. | |
Motion * | bestGoalMotion_ {nullptr} |
The best goal motion. | |
std::vector< Motion * > | goalMotions_ |
A list of states in the tree that satisfy the goal condition. | |
bool | useTreePruning_ {false} |
The status of the tree pruning option. | |
double | pruneThreshold_ {.05} |
The tree is pruned when the change in solution cost is greater than this fraction. | |
bool | usePrunedMeasure_ {false} |
Option to use the informed measure. | |
bool | useInformedSampling_ {false} |
Option to use informed sampling. | |
bool | useRejectionSampling_ {false} |
The status of the sample rejection parameter. | |
bool | useNewStateRejection_ {false} |
The status of the new-state rejection parameter. | |
bool | useAdmissibleCostToCome_ {true} |
The admissibility of the new-state rejection heuristic. | |
unsigned int | numSampleAttempts_ {100u} |
The number of attempts to make at informed sampling. | |
bool | useOrderedSampling_ {false} |
Option to create batches of samples and order them. | |
unsigned int | batchSize_ {1u} |
The size of the batches. | |
std::vector< Motion * > | startMotions_ |
Stores the start states as Motions. | |
base::Cost | bestCost_ {std::numeric_limits<double>::quiet_NaN()} |
Best cost found so far by algorithm. | |
base::Cost | prunedCost_ {std::numeric_limits<double>::quiet_NaN()} |
The cost at which the graph was last pruned. | |
double | prunedMeasure_ {0.} |
The measure of the problem when we pruned it (if this isn't in use, it will be set to si_->getSpaceMeasure()) | |
unsigned int | iterations_ {0u} |
Number of iterations the algorithm performed. | |
![]() | |
SpaceInformationPtr | si_ |
The space information for which planning is done. | |
ProblemDefinitionPtr | pdef_ |
The user set problem definition. | |
PlannerInputStates | pis_ |
Utility class to extract valid input states | |
std::string | name_ |
The name of this planner. | |
PlannerSpecs | specs_ |
The specifications of the planner (its capabilities) | |
ParamSet | params_ |
A map from parameter names to parameter instances for this planner. This field is populated by the declareParam() function. | |
PlannerProgressProperties | plannerProgressProperties_ |
A mapping between this planner's progress property names and the functions used for querying those progress properties. | |
bool | setup_ |
Flag indicating whether setup() has been called. | |
Additional Inherited Members | |
![]() | |
using | PlannerProgressProperty = std::function< std::string()> |
Definition of a function which returns a property about the planner's progress that can be queried by a benchmarking routine. | |
using | PlannerProgressProperties = std::map< std::string, PlannerProgressProperty > |
A dictionary which maps the name of a progress property to the function to be used for querying that property. | |
Detailed Description
Optimal Rapidly-exploring Random Trees.
- Short description
- RRT* (optimal RRT) is an asymptotically-optimal incremental sampling-based motion planning algorithm. RRT* algorithm is guaranteed to converge to an optimal solution, while its running time is guaranteed to be a constant factor of the running time of the RRT. The notion of optimality is with respect to a specified OptimizationObjective (set in the ProblemDefinition). If a solution path's cost is within a user-specified cost-threshold, the algorithm terminates before the elapsed time.
- External documentation
- S. Karaman and E. Frazzoli, Sampling-based Algorithms for Optimal Motion Planning, International Journal of Robotics Research, Vol 30, No 7, 2011. https://arxiv.org/abs/1105.1186
Member Function Documentation
◆ setGoalBias()
|
inline |
Set the goal bias.
In the process of randomly selecting states in the state space to attempt to go towards, the algorithm may in fact choose the actual goal state, if it knows it, with some probability. This probability is a real number between 0.0 and 1.0; its value should usually be around 0.05 and should not be too large. It is probably a good idea to use the default value.
◆ setRange()
|
inline |
The documentation for this class was generated from the following files:
- ompl/geometric/planners/rrt/RRTstar.h
- ompl/geometric/planners/rrt/src/RRTstar.cpp